Using Neural Networks for Growing Cucumbers
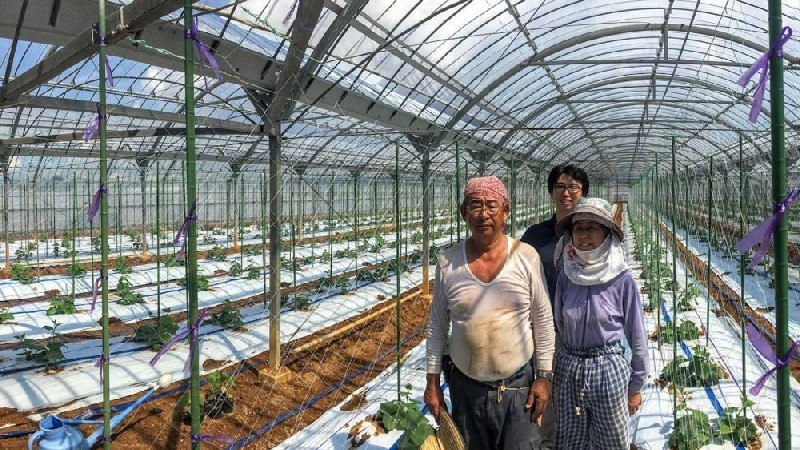
💡 Want more business insights? Stay ahead of the curve with our exclusive updates!
👉 Join our Telegram channel for daily business ideas and expert tips.
👉 Follow us on Facebook to never miss a trend or update!
Don’t just read—connect, grow, and innovate with us today!
The Advancements in Cognitive Systems Technology in Agriculture
The development and application of modern cognitive systems are constantly progressing. One recent example is the experiments conducted in agriculture by a young American engineer named Makoto Koike.
The Challenge
It all began with a simple problem. Makoto's parents are cucumber farmers. Sorting their final product adds complexity to an already challenging process. Large and uniform cucumbers fetch higher prices compared to their smaller, misshapen counterparts. This sorting task consumes a significant amount of time and labor for the farmers. To simplify this task, the American engineer utilized neural networks.
Technological Foundation
The sorting algorithm is based on Google's open-source development, TensorFlow. In 2015, the company open-sourced the technology, making it accessible for utilization in neural network applications.
The hardware involved Raspberry Pi 3 and Arduino Micro technologies. These components controlled the sorter and the camera, which sent images to Google Cloud for immediate analysis.
How It Works?
The device comprises a conveyor belt, a camera for snapshots, and a specialized mechanical arm that sorts cucumbers into baskets. As a cucumber moves along the belt, the camera captures images and sends them to the neural network, which then classifies them accordingly.
Prior to deployment, the engineer captured images of over 7000 vegetables. However, even this vast dataset was insufficient, resulting in the system achieving only 70% efficiency (compared to the initial 95% on preliminary tests). This is mostly attributed to:
1) The dataset of 7000 photos was inadequate for proper neural network training.
2) The low resolution of the photos (80x80 pixels) leads to the system missing finer details, thus reducing its efficiency. Increasing the image quality would significantly lengthen the network training time.
💡 Want more business insights? Stay ahead of the curve with our exclusive updates!
👉 Join our Telegram channel for daily business ideas and expert tips.
👉 Follow us on Facebook to never miss a trend or update!
Don’t just read—connect, grow, and innovate with us today!
Note: Comments are being moderated and may take a while to appear. There is no need to resubmit your comment.